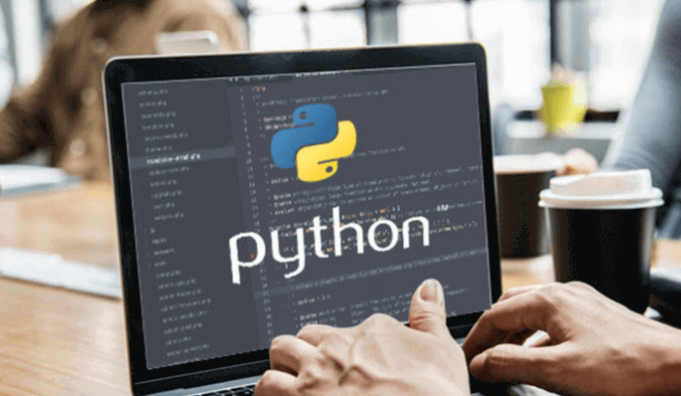
1. 파이썬 시작하기: 기본 설치 및 환경 설정
파이썬은 초보자부터 전문가까지 모두 사용하는 인기 있는 프로그래밍 언어입니다. 시작하기 전에, 먼저 파이썬을 어떻게 설치하고 설정하는지 알아봅시다.
설치하기
- 파이썬 공식 홈페이지(Python.org)에서 최신 버전의 파이썬을 다운로드 받을 수 있습니다.
- 다운로드 후 설치 과정에서 “Add Python to PATH” 옵션을 체크하면, 파이썬을 시스템 전체에서 쉽게 사용할 수 있습니다.
환경 설정
- IDE(Integrated Development Environment)는 파이썬 코드 작성에 도움을 줍니다. 인기 있는 IDE로는 PyCharm, Visual Studio Code 등이 있습니다.
- 필요한 라이브러리나 패키지는
pip
를 이용해 설치할 수 있습니다.
예시: 라이브러리 설치하기
pip install requests
2. 파이썬의 기본 문법: 변수, 데이터 타입, 조건문 및 반복문
파이썬은 문법이 간결하며 읽기 쉬워 많은 사람들이 선택하는 언어 중 하나입니다. 기본 문법을 잘 알아두면, 다양한 프로그램을 쉽게 작성할 수 있습니다.
변수와 데이터 타입
- 파이썬은 동적 타이핑 언어입니다. 따라서 변수 선언시 타입을 지정해주지 않아도 됩니다.
name = "John"
age = 30
조건문
조건에 따라 다른 코드를 실행할 때 사용합니다.
if age > 20:
print("성인입니다.")
else:
print("청소년입니다.")
반복문
동일한 작업을 여러 번 수행할 때 사용합니다.
for i in range(5):
print(i)
3. 파이썬으로 프로젝트 시작하기: 웹 크롤링
웹 크롤링은 웹사이트의 정보를 자동으로 추출하는 기법입니다. 파이썬은 BeautifulSoup
과 requests
라이브러리로 간단하게 웹 크롤링을 수행할 수 있습니다.
설치하기
pip install beautifulsoup4 requests
기본 예시
import requests
from bs4 import BeautifulSoup
URL = 'https://example.com'
response = requests.get(URL)
soup = BeautifulSoup(response.text, 'html.parser')
title = soup.title.text
print(title)
이 예시는 https://example.com
의 타이틀을 출력하는 간단한 크롤러입니다.
4. 파이썬의 데이터 처리: 리스트와 딕셔너리
데이터 처리는 프로그래밍에서 중요한 부분입니다. 파이썬은 다양한 자료형과 함께 기본적인 데이터 처리 기능을 제공합니다.
리스트(List)
- 순서가 있는 항목의 집합입니다.
- 대괄호([])로 표시하며, 아이템은 쉼표로 구분합니다.
fruits = ["apple", "banana", "cherry"]
fruits.append("orange")
print(fruits) # ['apple', 'banana', 'cherry', 'orange']
딕셔너리(Dictionary)
- 키와 값의 쌍으로 구성된 항목의 집합입니다.
- 중괄호({})로 표시합니다.
person = {
"name": "John",
"age": 30
}
print(person["name"]) # John
5. 파이썬의 함수와 클래스: 모듈화와 객체 지향 프로그래밍
코드를 재사용하고 구조화하는 데 도움을 주는 기능입니다.
함수(Function)
- 특정 작업을 수행하는 코드 블록입니다.
def
키워드를 사용하여 정의합니다.
def greet(name):
return "Hello, " + name + "!"
print(greet("Alice")) # Hello, Alice!
클래스(Class)
- 객체를 생성하기 위한 틀 또는 설계도입니다.
- 객체 지향 프로그래밍의 핵심입니다.
class Car:
def __init__(self, brand, model):
self.brand = brand
self.model = model
def display(self):
return f"This is a {self.brand} {self.model}."
my_car = Car("Toyota", "Corolla")
print(my_car.display()) # This is a Toyota Corolla.
6. 파이썬 라이브러리 활용하기: Numpy와 Pandas
데이터 분석과 과학 연산 작업에 파이썬 라이브러리는 필수입니다.
Numpy
- 대규모의 다차원 배열과 행렬 연산에 최적화된 라이브러리입니다.
import numpy as np
array = np.array([1, 2, 3, 4])
print(np.sum(array)) # 10
Pandas
- 데이터 분석을 위한 효과적인 데이터 구조를 제공하는 라이브러리입니다.
import pandas as pd
data = {
'names': ['Alice', 'Bob', 'Charlie'],
'age': [24, 30, 35]
}
df = pd.DataFrame(data)
print(df)
7. 웹 개발과 파이썬: Flask와 Django
파이썬으로 웹 애플리케이션을 개발하려면 Flask와 Django와 같은 프레임워크를 활용할 수 있습니다.
Flask
- 마이크로 웹 프레임워크로, 간단한 웹 애플리케이션을 빠르게 제작하는데 적합합니다.
from flask import Flask
app = Flask(__name__)
@app.route('/')
def hello():
return "Hello, World!"
if __name__ == '__main__':
app.run()
Django
- 풀 스택 웹 프레임워크로, 크고 복잡한 웹 애플리케이션을 구축하는데 적합합니다.
- ORM, 관리자 패널, 인증 등 많은 기능을 내장하고 있습니다.
# Django 프로젝트와 앱의 초기 설정 이후
from django.urls import path
from . import views
urlpatterns = [
path('', views.home, name='home'),
]
8. 데이터 시각화: Matplotlib와 Seaborn
데이터 분석 결과를 시각적으로 표현하려면 Matplotlib와 Seaborn을 활용할 수 있습니다.
Matplotlib
- 기본적인 그래프와 데이터 시각화 도구를 제공합니다.
import matplotlib.pyplot as plt
x = [1, 2, 3, 4]
y = [10, 20, 30, 40]
plt.plot(x, y)
plt.show()
Seaborn
- Matplotlib를 기반으로 한 고급 인터페이스를 제공하며, 보다 아름다운 그래프를 그릴 수 있습니다.
import seaborn as sns
tips = sns.load_dataset("tips")
sns.boxplot(x="day", y="total_bill", data=tips)
plt.show()
9. 머신러닝과 파이썬: Scikit-learn
머신러닝 모델을 쉽게 개발하고 훈련시키려면 Scikit-learn 라이브러리가 유용합니다.
from sklearn.ensemble import RandomForestClassifier
from sklearn.datasets import load_iris
from sklearn.model_selection import train_test_split
from sklearn.metrics import accuracy_score
data = load_iris()
X_train, X_test, y_train, y_test = train_test_split(data.data, data.target, test_size=0.3)
clf = RandomForestClassifier()
clf.fit(X_train, y_train)
predictions = clf.predict(X_test)
print(accuracy_score(y_test, predictions))
10. 딥러닝과 파이썬: TensorFlow와 PyTorch
딥러닝 알고리즘을 구현하고 훈련시키기 위한 라이브러리로 TensorFlow와 PyTorch가 대표적입니다.
TensorFlow
- Google이 개발한 오픈소스 라이브러리로, 간편한 API를 제공합니다.
import tensorflow as tf
mnist = tf.keras.datasets.mnist
(x_train, y_train), (x_test, y_test) = mnist.load_data()
x_train, x_test = x_train / 255.0, x_test / 255.0
model = tf.keras.models.Sequential([
tf.keras.layers.Flatten(input_shape=(28, 28)),
tf.keras.layers.Dense(128, activation='relu'),
tf.keras.layers.Dropout(0.2),
tf.keras.layers.Dense(10)
])
PyTorch
- Facebook이 개발한 딥러닝 라이브러리로, 연구자들 사이에서 인기가 높습니다.
import torch
import torch.nn as nn
import torch.optim as optim
class SimpleNN(nn.Module):
def __init__(self):
super(SimpleNN, self).__init__()
self.layers = nn.Sequential(
nn.Flatten(),
nn.Linear(28*28, 50),
nn.ReLU(),
nn.Linear(50, 10)
)
def forward(self, x):
return self.layers(x)
11. 웹 스크레이핑과 파이썬: BeautifulSoup과 Scrapy
인터넷에서 데이터를 수집하려면 파이썬의 웹 스크레이핑 도구들이 큰 도움을 줍니다.
BeautifulSoup
- 웹 페이지의 HTML과 XML을 파싱하기 위한 도구입니다.
- 간단한 스크레이핑 작업에 적합합니다.
from bs4 import BeautifulSoup
import requests
URL = "https://example.com"
response = requests.get(URL)
soup = BeautifulSoup(response.content, 'html.parser')
title = soup.title.string
print(title)
Scrapy
- 웹 크롤링 프레임워크로, 대규모 웹 스크레이핑 작업에 적합합니다.
import scrapy
class MySpider(scrapy.Spider):
name = 'my_spider'
start_urls = ['https://example.com']
def parse(self, response):
title = response.xpath('//title/text()').get()
self.log(f"Scraped title: {title}")
12. 데이터베이스와 파이썬: SQLite, SQLAlchemy
데이터 저장과 관리에는 데이터베이스가 필수적입니다. 파이썬은 다양한 데이터베이스 라이브러리를 지원합니다.
SQLite
- 경량의 파일 기반 데이터베이스입니다.
- 별도의 서버 설정 없이 사용 가능합니다.
import sqlite3
conn = sqlite3.connect('example.db')
c = conn.cursor()
c.execute('''CREATE TABLE stocks (date text, trans text, symbol text, qty real, price real)''')
c.execute("INSERT INTO stocks VALUES ('2023-08-27', 'BUY', 'AAPL', 100, 132.45)")
conn.commit()
conn.close()
SQLAlchemy
- SQL 툴킷 및 객체 관계 매핑(ORM) 라이브러리입니다.
- 다양한 데이터베이스 시스템과 연동이 가능합니다.
from sqlalchemy import create_engine, Column, Integer, String, Sequence
from sqlalchemy.ext.declarative import declarative_base
Base = declarative_base()
class User(Base):
__tablename__ = 'users'
id = Column(Integer, Sequence('user_id_seq'), primary_key=True)
name = Column(String(50))
engine = create_engine('sqlite:///:memory:')
Base.metadata.create_all(engine)
13. 병렬 및 비동기 프로그래밍: Threading, Asyncio
효율적인 프로그램 실행을 위해 병렬 및 비동기 처리를 사용할 수 있습니다.
Threading
- 파이썬에서 병렬 작업을 수행하는 기본 방법입니다.
import threading
def print_numbers():
for i in range(10):
print(i)
def print_letters():
for letter in 'abcdefghij':
print(letter)
t1 = threading.Thread(target=print_numbers)
t2 = threading.Thread(target=print_letters)
t1.start()
t2.start()
t1.join()
t2.join()
Asyncio
- 파이썬의 비동기 프로그래밍 프레임워크입니다.
import asyncio
async def say_hello():
await asyncio.sleep(1)
print("Hello")
async def say_world():
await asyncio.sleep(1)
print("World")
async def main():
await asyncio.gather(say_hello(), say_world())
asyncio.run(main())
파이썬은 그 강력함과 다양성으로 많은 분야에서 사용됩니다.
위의 주제들은 파이썬의 광범위한 영역 중 일부에 불과하니,
지속적인 학습과 연습을 통해 파이썬의 풍부한 기능을 최대한 활용해보세요!